By Crystal Taggart
The Promise and Pitfalls of AI in Medicine
A recent JAMA report revealed that chatGPT provided inaccurate diagnoses a startling 80% of the time across a 100 sample pediatric cases. This alarming result highlights a crucial distinction between raw conversational models versus AI developed specifically for clinical contexts. Before relying on AI tools in sensitive domains like healthcare, rigorous validation, testing, and clinical expertise is still pivotal.
The launch of chatGPT by OpenAI has sparked intrigue – and controversy – around AI’s growing capabilities for conversation and reasoning. Its surprisingly human-like responses have many anticipating AI’s potential in spheres like healthcare. However, while conversational tools like chatGPT preview the art of the possible, they lack the rigor required for reliable real-world medical applications.
The Risks of Conversational AI for Diagnosis
Unlike technologies designed expressly for the complexity of medical diagnosis, chatGPT is fundamentally conversational. Under the hood, it possesses no concept of medicine or human health – no way to model disease progression, analyze risk factors or symptoms, or weigh diagnostic hypotheses. Instead, it relies on probabilities learned from ingesting huge volumes of online text data. While this enables surprisingly coherent discussions, it lacks true understanding of what it’s recommending.
So when posed patient cases and asked for diagnostic and treatment suggestions, its responses may sound impressively on-target but lack meaningful reliability. The report found its suggestions often aligned with common initial hypotheses doctors might pursue, consistent with chatGPT’s pattern-recognition capabilities. However, without a robust medical framework mapping symptoms to likely underlying conditions, its diagnoses quickly become untrustworthy guesses. They may neglect key areas, fail to recognize atypical presentations, or ignore relevant risk factors.
Doctors refine initial hypotheses over time by integrating new patient details, considering unlikely diagnoses, and evolving their approach based on response to treatment. In contrast, chatGPT has no mechanism to course-correct or account for the progression of disease and complexity of the diagnostic process. It isn’t integrating the dialogue history, patient medical records, test results, or any understanding of pathophysiology. This reliance on surface-level pattern matching means high potential for oversight, bias, and error.
So while the technology may someday contribute to projects like medical learning and education, it cannot replace human clinicians, particularly for rendering diagnoses. In fact, chatGPT itself cautions users against relying on its medical advice for health issues. Unvalidated AI tools recommending diagnoses creates dangerous potential for misdiagnosis and inappropriate treatment.
The Need for Rigor in Medical AI Tools
If chatGPT isn’t up to the task of diagnosis, where does the promise of AI-assistance come in? In a word: validation.
Rather than raw conversational models, reliable medical applications require purpose-built AI that specialists design expressly to address the nuances of clinical care. This includes technologies like AdviNOW’s diagnostic engine, developed by doctors alongside industry experts in AI and pathology.
Far from a generalist model, these tools undergo meticulous training, validation, and feedback cycles from practitioners. The models are customized to relevant diagnostic tasks using proven techniques in computer vision, predictive algorithms, and pattern recognition. By specializing around clinical workflows, the tools seamlessly integrate with provider diagnostic processes versus presenting standalone guesses.
For instance, AdviNOW’s software takes patient risk factors, demographics, symptoms and more as inputs – key contextual details chatGPT lacks. Drawing on aggregated learnings from patient cases, it surfaces clinically-relevant diagnostic suggestions consistent with the latest medical evidence and best practices. Both likely and unlikely diagnoses are highlighted to minimize oversight and human bias, helping to inform clinician intuition rather than replacing it.
Importantly, recommendations must pass expert standards before ever reaching physicians and patients. Evaluating diagnostic performance typically involves assessing parameters like consistency, accuracy relative to final diagnoses, and coverage of relevant alternatives. Models undergo audits by doctors who probe boundary cases, evaluate real-world usage, and submit diagnostic stumpers to further strengthen reliability.
These experiential feedback cycles enable the AI to continually learn – self-correcting errors, retaining new insights, and staying current with the latest research. This emphasis on rigorous clinical verification starkly contrasts the “proceed at your own risk” nature of chatGPT-style tools today.
Integrating AI into Clinical Workflows
Beyond accuracy, seamless integration with clinical workflows is also pivotal for practical application of AI diagnostics – another area where chatGPT falls short. Conversational tools lack mechanisms to intake relevant patient details, incorporate diagnostic history, connect recommendations with downstream care, or discern risk factors that could refine suggestions over time.
Purpose-built diagnostic aides, on the other hand, plug directly into electronic medical records (EMRs) when permitted. This allows automatically surfacing relevant background, gathering details providers documented, and refining recommendations as new signals emerge. Rather than a standalone guess, physicians can assess inferences alongside the patient story.
Some tools also allow validating the rationale behind conclusions, keying into the model’s reasoning process. This transparency helps synthesize outputs versus seeing suggestions as black-box guesses.
Over time, capturing predictive performance compared to final diagnoses further tunes reliability – a level of accountability uncommon in consumer tools like chatGPT today. Logging performance also informs model updates, allowing perpetual improvement from experience.
Lastly, integrating diagnostic AI with clinical systems keeps the patient-provider loop unbroken. Recommendations reached in error undergo timely revision by doctors close to each case. And revisiting guidance against patient history streamlines future decisions tailored to the individual.
The Data Privacy Dimension
As tools eyed for healthcare undergo evaluation, data privacy implications also warrant consideration. When applied to sensitive patient information, protecting confidentiality becomes even more paramount.
As a publicly accessible tool still in research phases, chatGPT does not yet meet healthcare’s stringent security and compliance standards. Without enterprise controls, mechanisms like access restrictions, audit logs, and heightened cybersecurity controls understandably remain lacking.
Consumer models also inform the platform’s continuous training. By policy, OpenAI reserves rights to ingest queries users submit to further teach its models – concerning for those who inadvertently opt-in people by using chatGPT for medical advice. And while information likely undergoes scrubbing beforehand, potentially traceable imprints still lurk.
So beyond lacking contextual reliability to inform diagnoses, chatGPT’s privacy approach also falls short of healthcare’s regulated protections – unlike clinically-validated tools. AdviNOW’s diagnostic engine underwent HIPAA compliance certification to address the privacy and security requirements involved in processing patient data. And strict access controls ensure only verified medical professionals interface with sensitive information to safeguard patients.
AI’s Future in Medicine
ChatGPT and similar conversational tools certainly remain remarkable achievements, demonstrating AI’s burgeoning potential for creativity and reasoning. Their ability to discuss complex topics and fields could undoubtedly augment applications in medical learning and education someday. However, substantial rigor remains imperative before these technologies intervene in high-stakes healthcare decisions.
Specialized, clinically-validated AI like AdviNOW’s diagnostic engine points to the future:
Purpose-built for practitioners rather than the public. Meticulously-trained on the latest medical evidence rather than gleaned from online mimicry. Seamlessly integrated with clinical workflows instead of presenting back-of-napkin guesses. Supporting clinicians with transparent probabilities versus attempting to replace human judgment.
Getting medical AI right mandates focus. Beyond raw technological capability, responsibly translating tools into patient impact requires diligent clinical assurance, prizing safety over novelty, and enabling human practitioners to exercise their well-honed expertise. If effort concentrates on empowering doctors rather than impressing casual users, medical AI’s immense potential still clearly shines through.
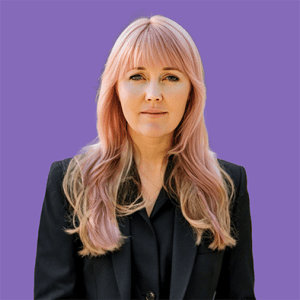
Crystal Taggart Head of Innovation at AdviNOW
About the Author
Crystal Taggart is currently the Head of Innovation at AdviNOW Medical. Her journey in technology spans over 25 years, encompassing diverse roles in software development, IT strategy, and digital transformation. Crystal holds an MBA from Eller School of Management, fueling her expertise in creating cutting-edge tech solutions in the healthcare sector.